Talk to almost anyone — anyone human, that is — and your conversation will encounter what Malihe Alikhani calls “healthy frictions,” moments where your goal in the conversation bumps up against your partner’s, moments that require clarification, produce confusion or lead to disagreement.
Not so with large language models.
Alikhani, an assistant professor in the Khoury College of Computer Sciences at Northeastern University, says that large language models like ChatGPT have a serious problem with certainty.
Alikhani’s new project, called Friction for Accountability in Conversational Transactions (FACT), is a collaboration between Northeastern University, the University of Illinois Urbana-Champaign and the University of Southern California.
Funded through an Artificial Intelligence Exploration grant through the Defense Advanced Research Project Agency, the FACT project aims to develop more transparent and equitable artificial intelligence tools.
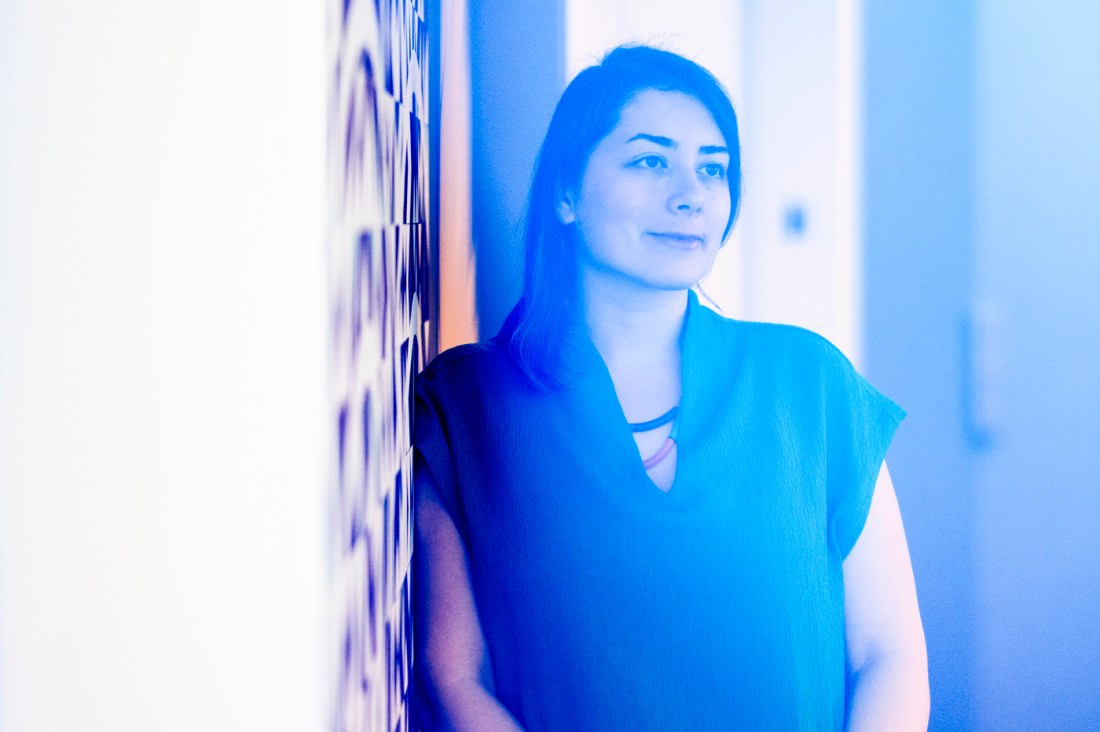
“One of the things that makes human communication a fruitful tool,” Alikhani says, “is the fact that we represent our uncertainty in our speech, in our tone. We put it in our facial expression.”
The healthy frictions that arise from uncertainty in human-to-human communication help maintain a diversity of opinions and viewpoints, she continues.
But large language models (or LLMs) aren’t interested in expressing their uncertainty, resulting in what Alikhani calls “sycophantic behaviors.” Large language models “want to maximize the satisfaction” of their user, she says, and “never introduce any friction in the conversation, whether [the model is] confident” of its statements or not.
Additional problems arise with large language models through their tendency to hallucinate. LLMs “make up facts. They are very good at persuading people of facts that are made up.”
Despite these issues, Alikhani also says that humans are prone to over relying on the “facts” generated by these artificial intelligence models, which “may make up facts to make you happy.”
Part of what contributes to user overreliance on LLMs are their “human-like behaviors,” she says. “That will manipulate our cognition.”
Large language models also seem to produce their responses instantaneously, another factor that makes users assume correctness. “It’s hard for us AI scientists to tell people, ‘Yeah, it’s coherent. Yes, it is fast. Yes, it’s tuning into your style. But it hallucinates,’” Alikhani says.
Under their new grant, Alikhani and her team will design tools that demonstrate the levels of certainty an LLM holds about a statement it makes and introduce healthy frictions into human-AI conversations.
“How can we predict and verbalize the confidence of the system?” Alikhani asks. If an AI model is “only 2% confident, it should externalize that.”
“One of the main goals of the research is to model uncertainty, to externalize uncertainty” and teach LLMs how to portray that uncertainty within a human-AI conversation. This might appear in a user’s interface as a percentile score of the model’s certainty, or the model might reflect uncertainty in its responses in a more human-like way.
For instance, Alikhani imagines a situation in which a patient might ask a large language model a question about their health. The current generation of LLMs will try to provide an answer, even if that answer might turn out to be dangerous. Alikhani hopes to build models that can say, “‘I don’t know. You should call your nurse.’”
“Robustness is key to accountability in AI,” Alikhani says. At the moment, it’s common for an LLM to respond with one answer to a query at the time of asking and a completely different answer a few minutes later.
When it comes to designing AI that’s both safe and accountable, previous AI systems that might help with simple tasks “didn’t have access to a bunch of other datasets,” Alikhani says, “and they couldn’t say things that might be dangerous, because it was not in their data.”
Exactly what those datasets include — or exclude — are key to overcoming the biases LLMs display toward “gender, but also subtler biases, such as in- versus out-groups and different cognitive biases that are reflected in [large language] models.”
Now, Alikhani hopes to design models that service people with “different affordances and preferences,” she says.
“We don’t want to just keep building systems for the population we have data for, but we think about who we are leaving behind, and how can we stop this huge gap of inequality instead of making it worse?” she asks. “The goal of my lab is to move towards that direction.”
Noah Lloyd is a Senior Writer for NGN Research. Email him at n.lloyd@northeastern.edu. Follow him on X/Twitter at @noahghola.